If there was one certainty in a world after Brexit, it was that the United States was poised to elect its first female president, and in doing so return some stability to the emerging global nationalistic tumult.
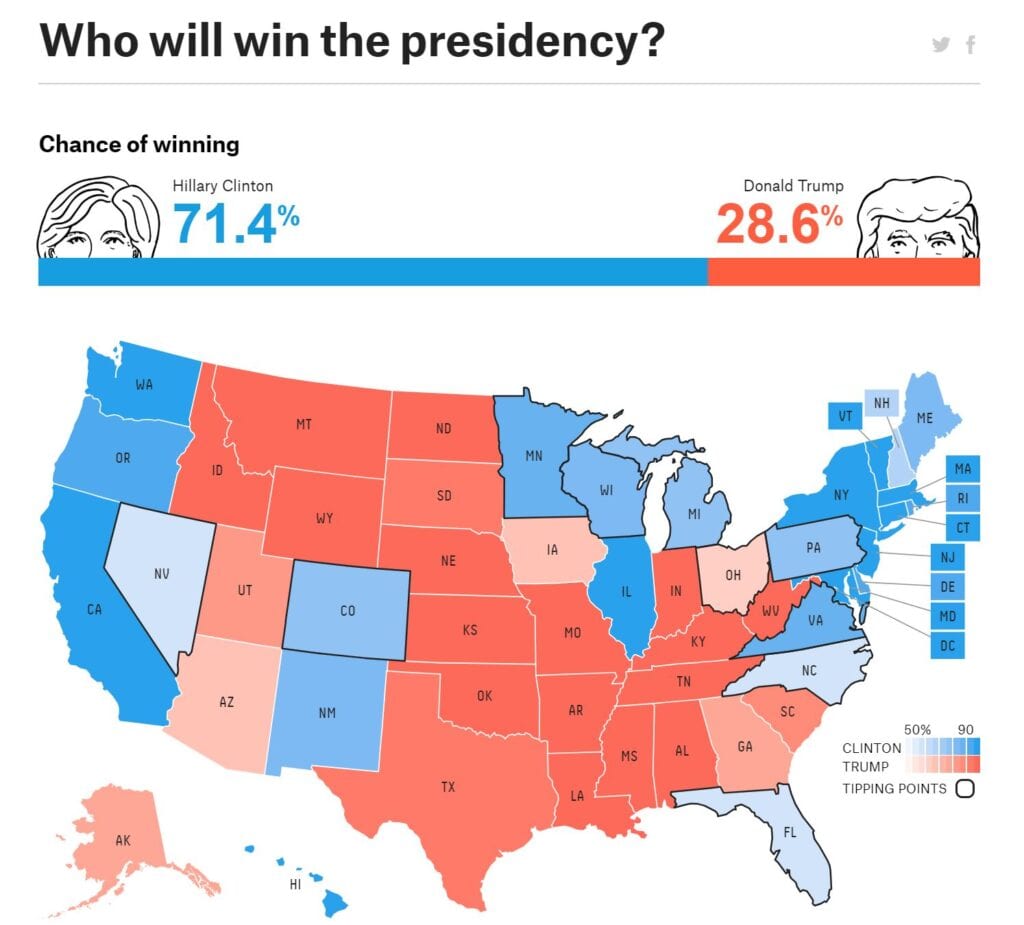
But on the evening of November 8, 2017, the American electorate proved nearly every analyst wrong as Donald Trump received a higher number of electoral votes, and with that the new title of president-elect.
For the rest of the night, and the weeks and months that followed, pundits and talking heads rationalized their inaccurate predictions. It was as painful to watch as it was to endure.
The recent allegations against Cambridge Analytica brought these concerns back to the surface. Russia. Cambridge Analytica. James Comey. Nearly 18 months later, analysts are still looking to lay blame with any outside force that could have distorted their data.
The problem isn’t that the data lied. The problem is that people do.
Big data is inherently objective. The computers that compile the data aren’t susceptible to bias, but both the individuals polled and the analysts interpreting the data are intrinsically biased. The mistake was underestimating confirmation bias and the human factor.
So, yes, big data is theoretically an improvement over data samples, but it is still imperfect. Voters have plenty of reason to conceal certain opinions. The fear of getting “found out” can be a powerful motivator. It’s easier to claim to be “undecided” than to publicly or privately acknowledge support for the demonized choice in your social sphere.
Besides, when the president normalizes lying, should it be all that surprising when his constituents follow suit?